News
NEWS
Latest news about Incenda AI and a collection of articles about the importance of data quality.
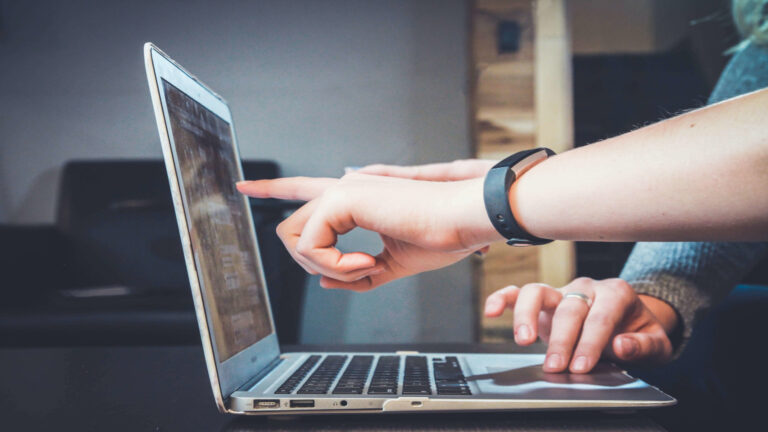
Incenda AI nun Teil der vom BMBF initiierten Plattform Lernende Systeme
Published on Aug 16, 2022 by Plattform Lernende Systeme, acatech
“Die Plattform Lernende Systeme ist ein vom BMBF initiiertes und bei acatech angesiedeltes Netzwerk von Expertinnen und Experten zum Thema Künstliche Intelligenz mit knapp 200 Mitgliedern aus Wissenschaft, Wirtschaft und Gesellschaft. Ziel ist es, den interdisziplinären Austausch sowie den gesellschaftlichen Dialog zu Entwicklung und Einsatz von KI-Systemen zu fördern.”
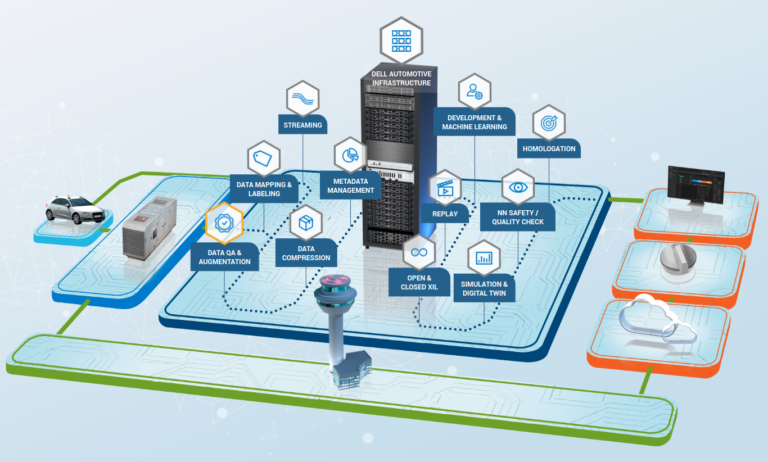
Dell Technologies Autonomous Drive Ecosystem
Published on June 17, 2022 by Marius Reuther, CEO at Incenda AI
We have partnered with Dell Technologies as a part of a comprehensive, open partner ecosystem for Advanced Driver Assistance Systems / Autonomous Driving (ADAS/AD) development. Incenda AI is part of an ecosystem of vendors selected as best-in-class for ADAS/AD test and development.
This new 3D interactive experience depicts everything from the connected Edge, to a vision of the expansive data pipeline that will be required to enable connected vehicles from the Edge to Core to Cloud, and the ecosystem of automotive AI & software providers that Dell is working with.
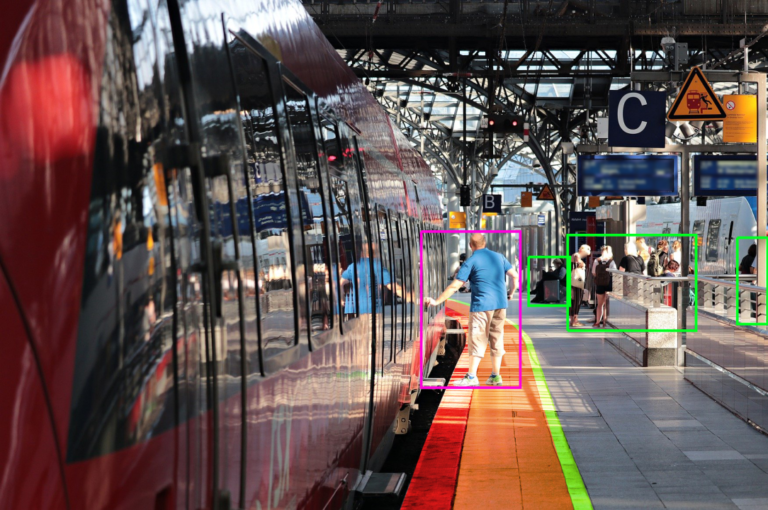
Is more always better ?
Published on July 12, 2021 by Max Ronecker, Machine Learning Engineer at Incenda AI
In Machine Learning the mantra for many years has been: more data equals better results! And to be honest in most cases this holds true.
Datasets have been growing from several thousand data-points to now billions and billions with a tendency to grow even larger. However getting evermore data brings its own set of challenges. Training time increases, data collection can be tedious and expensive, especially for very specific domains. But data is complex and not all is equal.
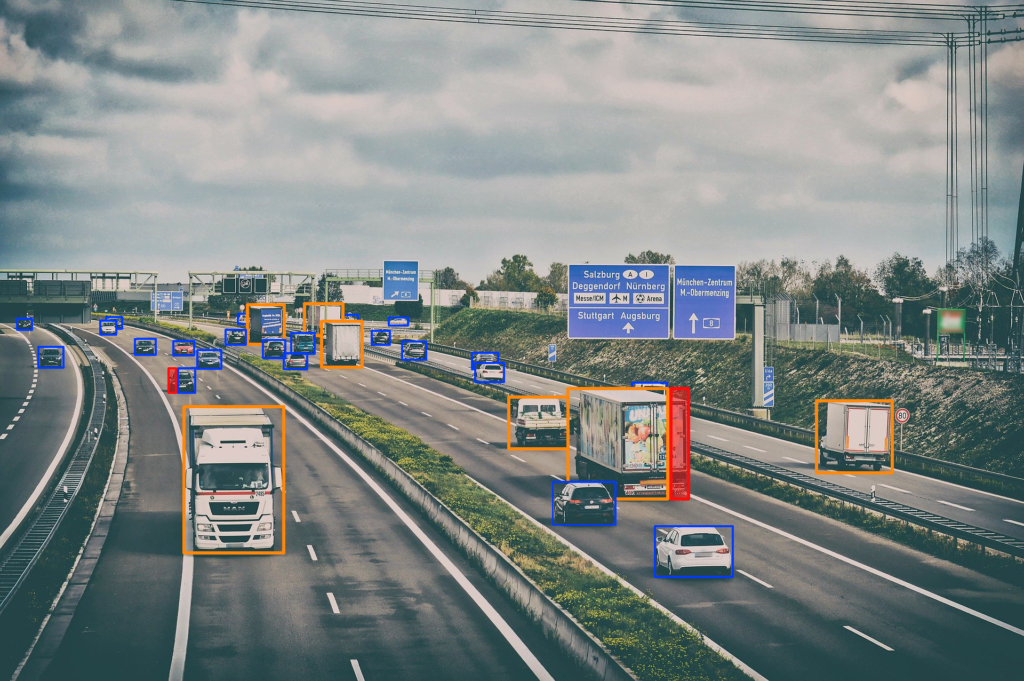
About what mean average precision is hiding
Published on April 19, 2021 by Max Ronecker, Machine Learning Engineer at Incenda AI
A variety of metrics exist in the area of machine learning. There are the classics like recall, precision and f1- score and the more exotic ones like Normalized Discounted Cumulative Gain. Whatever application you are trying to develop there is probably already a metric in place to evaluate it. Hence, it is difficult to have a thorough understanding of all of them.
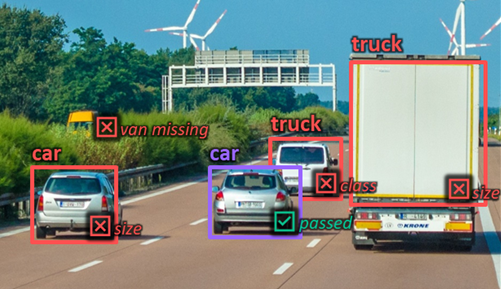
Part 2: The importance of data quality
Published on March 25, 2021 by Max Ronecker, Machine Learning Engineer at Incenda AI
In Part 1 of this series our partner from Lightly had a look at what redundancy in data does to your machine learning model. In this part we deal with the question what impact Issues in your labeling can have on machine learning performance.
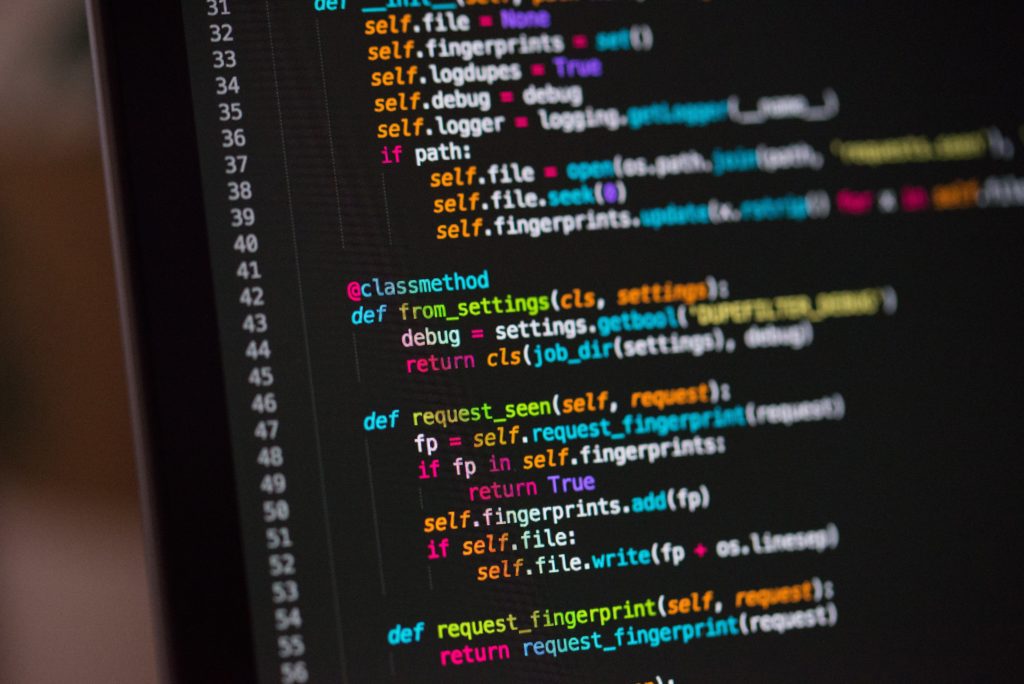
Part 1: The importance of data quality
Published on March 18, 2021 by Igor Susmelj, Co-Founder Lightly AI
Did you know that popular datasets such as ImageNet and Kitti contain redundant data? Learn more about data redundancy, how it can harm your machine learning model performance and what you can do against it.
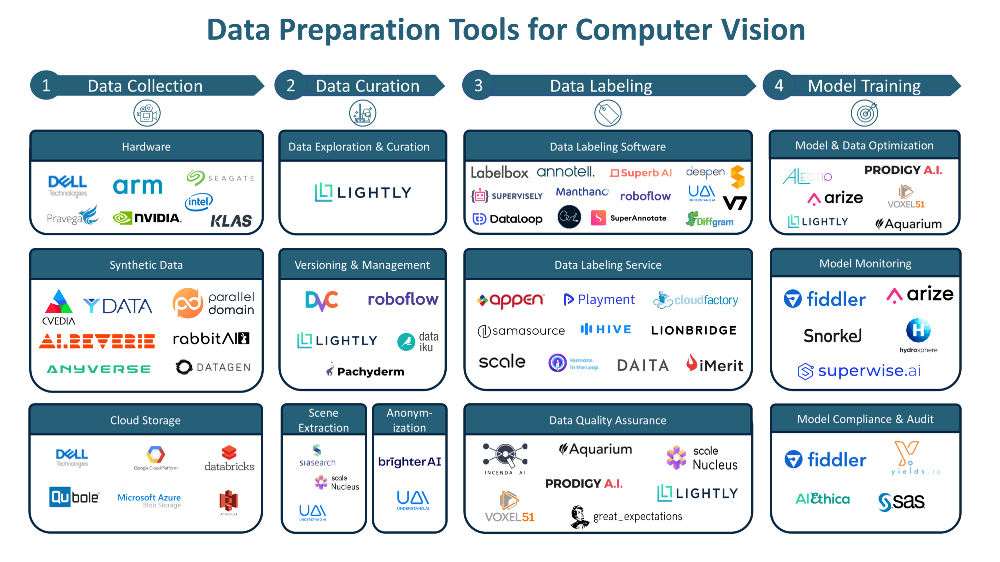
Data Preparation Tools for Computer Vision 2021
Published on March 18, 2021 by Matthias Heller, Co-Founder at Lightly AI
This article provides a data preparation tool landscape for computer vision 2021. The intention is to give an overview of the available solutions which machine learning engineers can use to build better models and be more efficient. Each segment is explained in detail below.
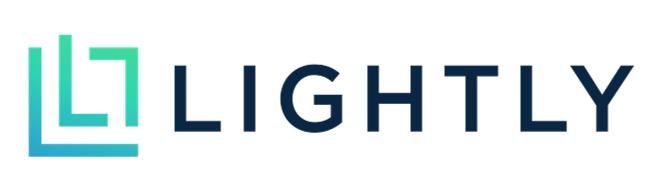
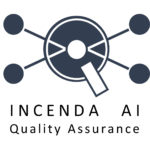
Partnership with Lightly
March 17, 2021
Building a business is a lot about focus, strategy & valuable partnerships.
Concentrate 120% on the core business, have a clear strategy for the future and team up with strong partners complementing own solutions is Incenda AI’s philosophy to do better than our competitors.
We appreciate to announce our partnership with Lightly. While focusing on the actual value of raw data Lightly shares our vision to highlight the importance of high-quality data for the AI development.
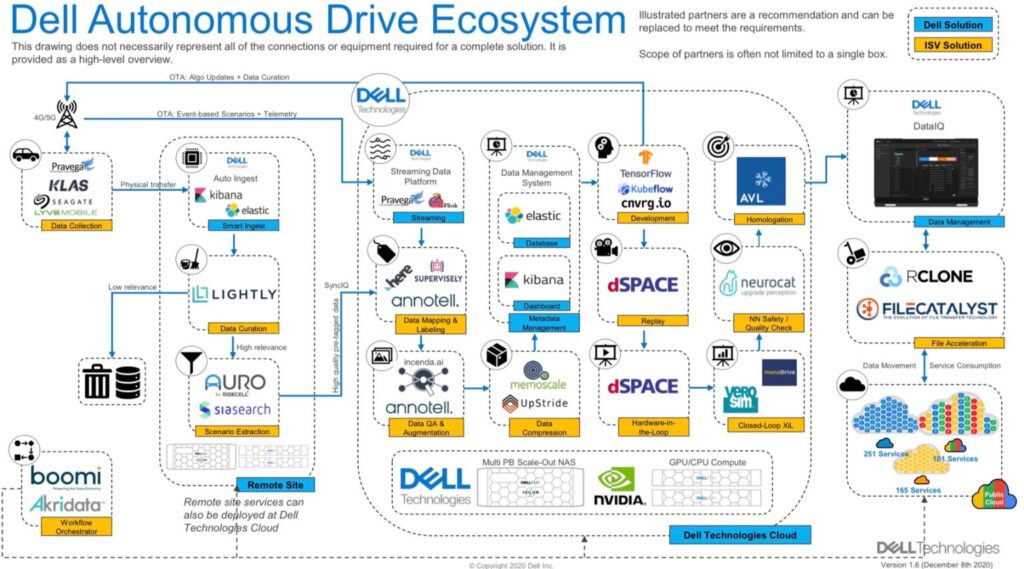
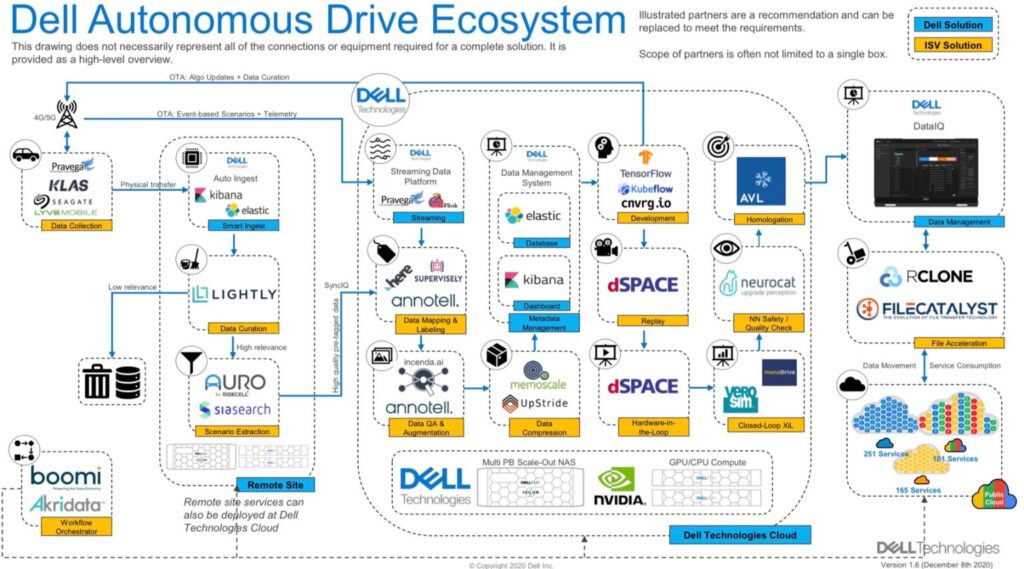
Dell Autonomous Drive Ecosystem
December, 2020 by Florian Baumann, CTO – Automotive & AI at Dell Technologies
It highlights the typical steps in an AD/ADAS workflow.
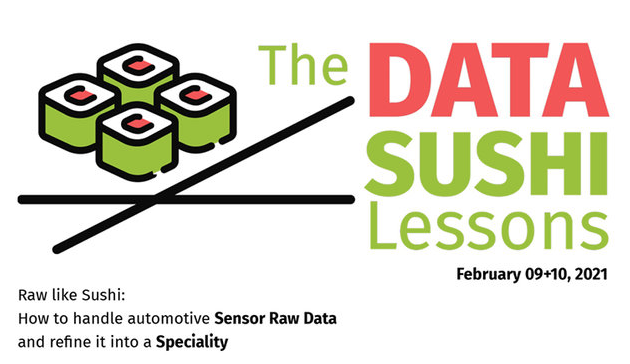
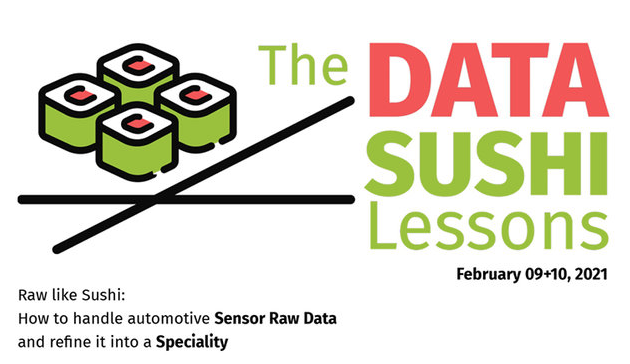
Recordings der Data Sushi Lessons online
March 4, 2021 by b-plus
Roh wie Sushi. Wie man automotive SENSOR-ROHDATEN erfasst und zu einer SPEZIALITÄT veredelt.
In der Webinarreihe “The Data Sushi Lessons” wurde dies von b-plus gemeinsam mit den Partnern Bertrandt, Deutronic, IBM, Incenda AI und Zukunft Mobility (ZF) gezeigt.
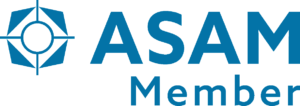
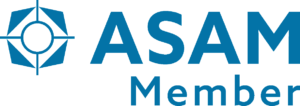
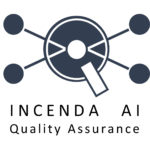
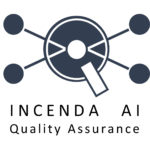
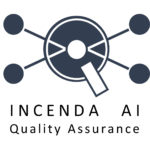
Membership with ASAM
December 14, 2020
OpenLABEL (final name still to be determined) aims to introduce a standardized set of labels for objects of interest (as identified by e.g. vehicle sensors) and for driving scenarios. In parallel it will define a generic labeling format applicable to data not specific to the two aforementioned use cases.
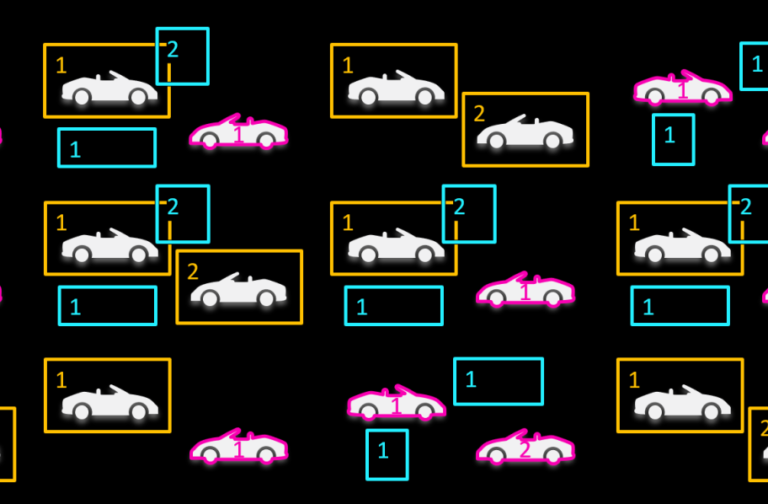
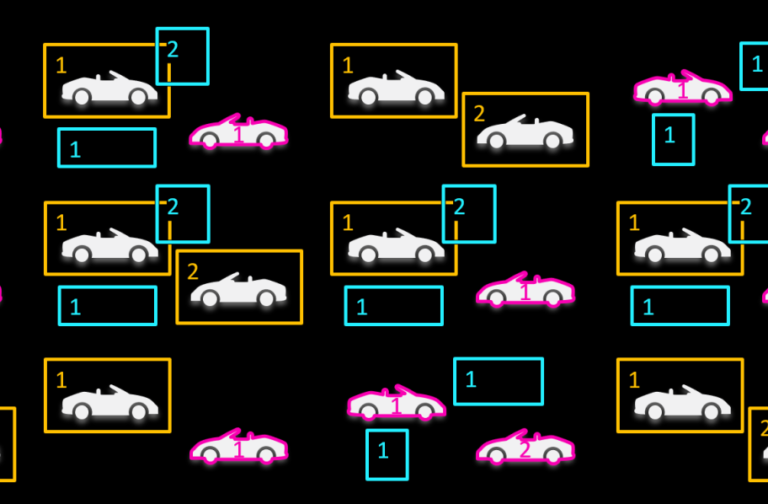
Part 3: Precision, Recall, F1-Score for Object Detection - Back to the ML Basics
Published on November 19, 2020 by Felix Friedmann, Co-Founder Incenda AI
There are some topics that we come across again and again. As Christoph Petzinger, a fellow (fantastic) software engineer, and me realized when we were back at camera interfacing for the n-th time, it may feel like walking a full circle, although every time you’ll be faster, better prepared.
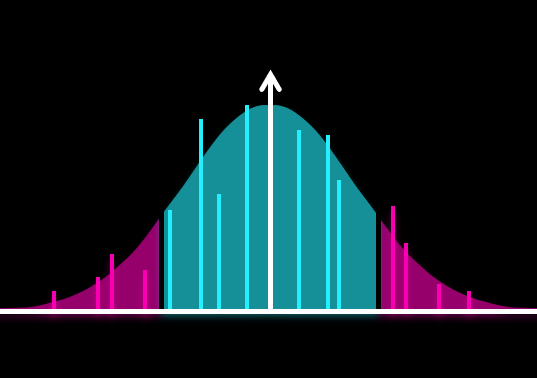
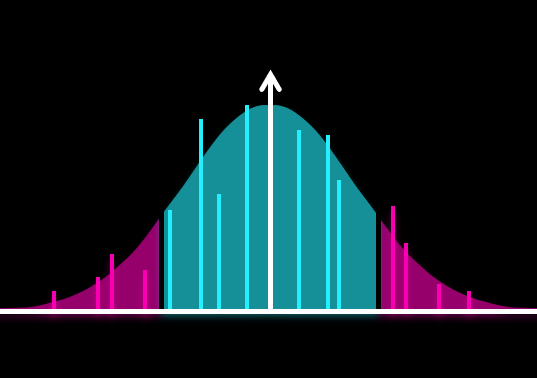
Part 2: Sample Size Determination for Data Quality Checks
Published on November 12, 2020 by Felix Friedmann, Co-Founder Incenda AI
After having recently discussed how to Fix (parts of) your Labeled Dataset, let’s now look into how we can assess the quality level of a dataset. For the metric we can assume a pass/fail outcome per data point in the dataset, based on e.g. thresholds on IoU, mAP, F1, etc. – will be covered in a separate article.
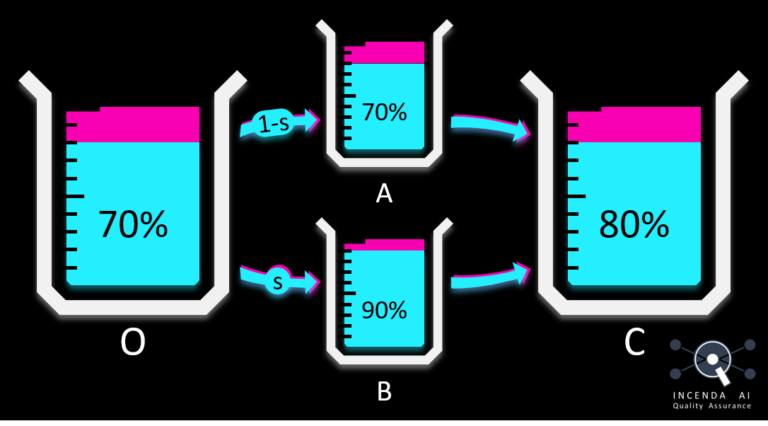
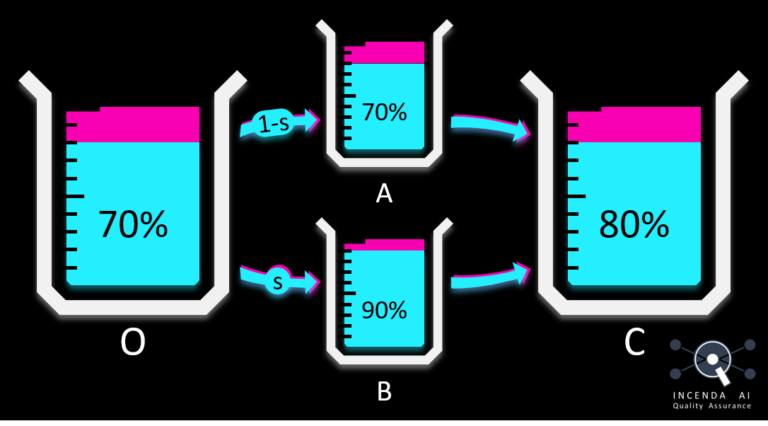
Part 1: Fixing (parts of) your Labeled Dataset
Published on October 28, 2020 by Felix Friedmann, Co-Founder Incenda AI
Supervised learning, i.e. training machine learning algorithms with annotated data, dominates commercial AI applications. This has led to tremendous pain for developers, who struggle with having their data collected, cleaned and labeled before they can even start to work on actual development.
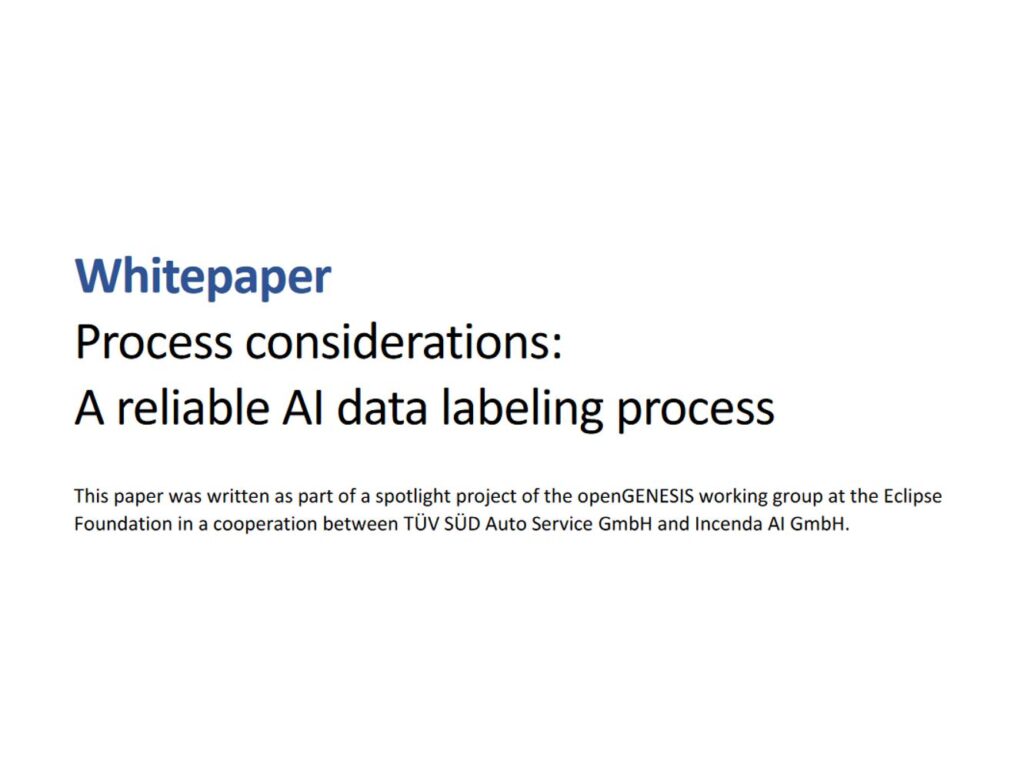
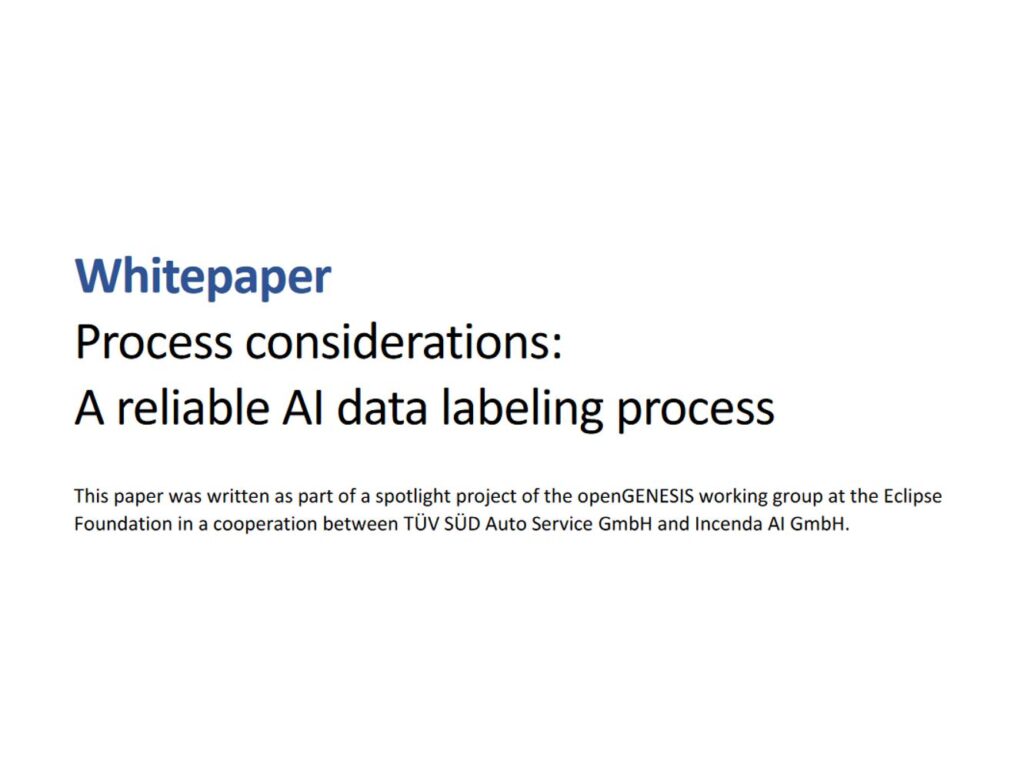
Process considerations: A reliable AI data labeling process
Published on October 21, 2020 by Incenda AI & TÜV SÜD
Incenda AI and TÜV SÜD collaborated to come up with a whitepaper: “A reliable AI data labeling process”.
This is needed as, so far, there is not much in terms of (quality) standards for data used to train AI models. We propose a Machine Learning Lifecycle and a Reference Labeling Process and discuss how they should be implemented to enable high data quality.
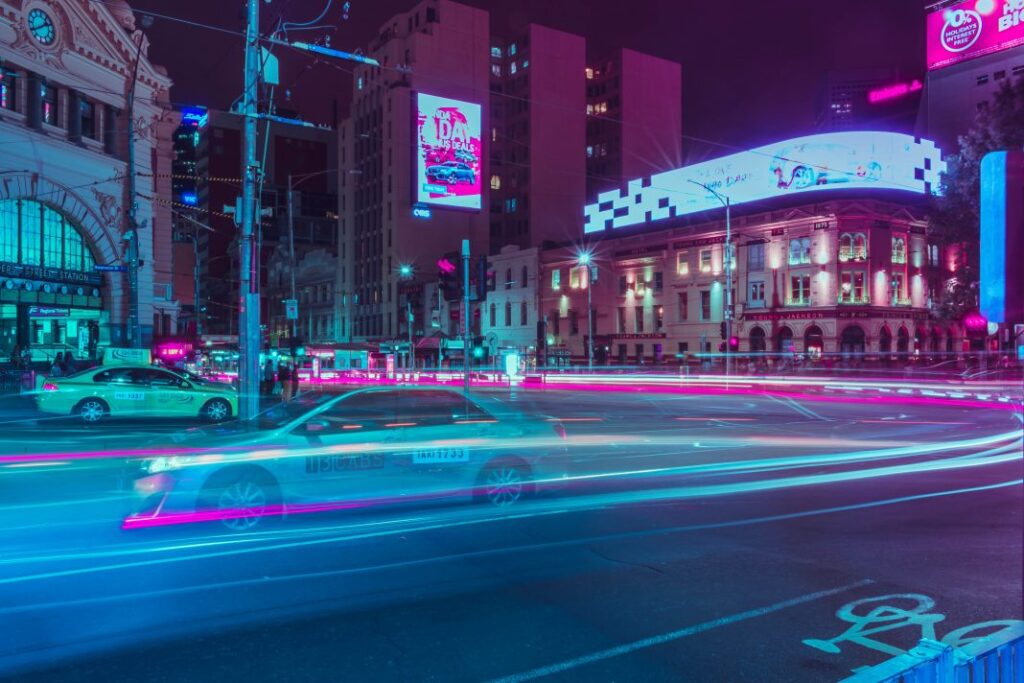
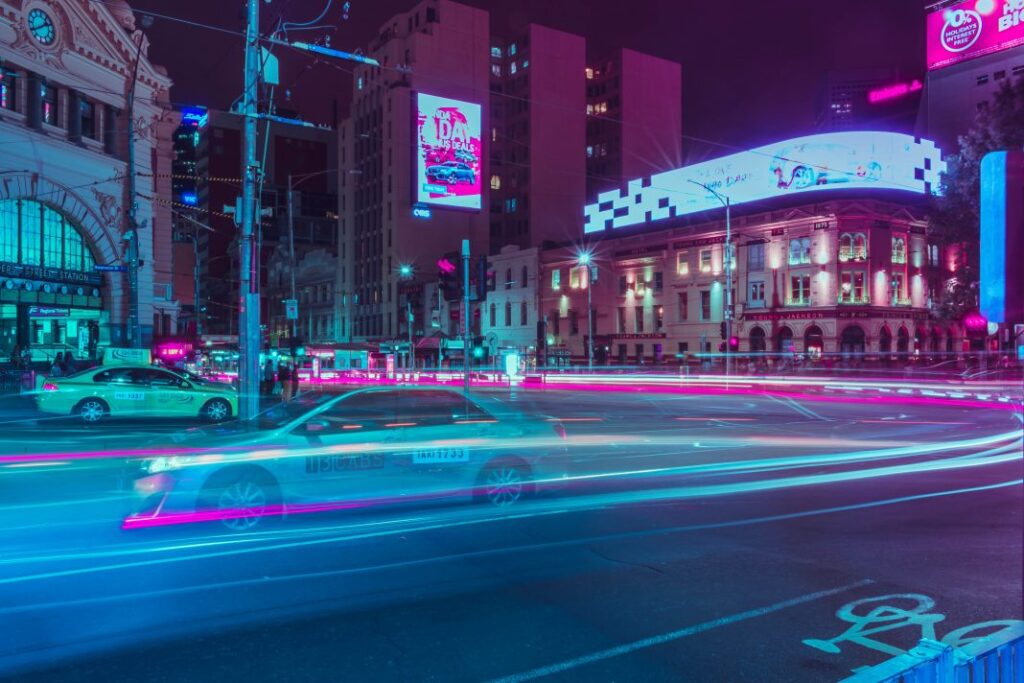
Autonomes Fahren beginnt im Rechenzentrum
Published on October 19, 2020 by Toan Nguyen
Unsere Mobilität muss und wird sich ändern, unsere Städte gelangen mit dem zunehmenden Individualverkehr an ihre Grenzen, und die ehrgeizigen Ziele im Bereich Klimaschutz lassen sich nur mit innovativen, nachhaltigen Konzepten erreichen.


Data Garage
Published on August 24, 2020
Autonomous driving is really an enormous challenge. Technologically one essential point, it is necessary to train the car with real environment data, which needs to be collected, transferred, pre-processed and stored beforehand. This process requires a complex chain of technologies from different providers.
Read the whitepaper or watch the webcast to learn more about the Data Garage project.